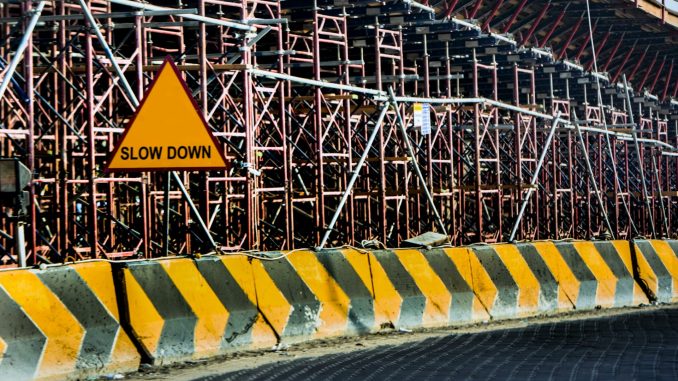
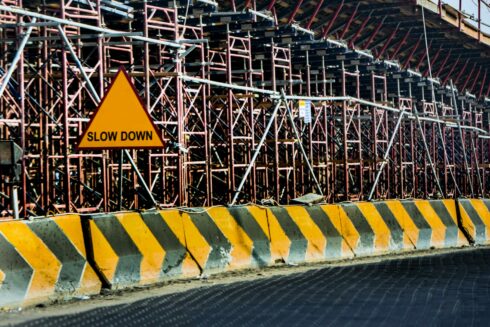
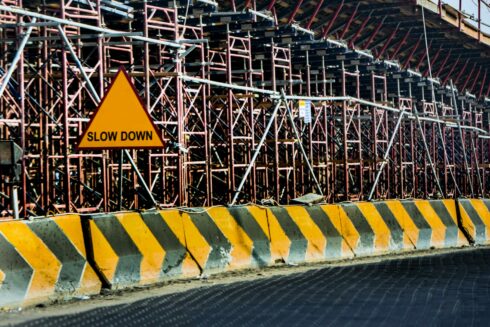
As artificial intelligence advances at breakneck speed, using it safely while also increasing its workload is a critical concern. Traditional methods of training safe AI have focused on filtering training data or fine-tuning models post-training to mitigate risks. However, in late May, Anthropic created a detailed map of the inner workings of its Claude 3 Sonnet model, revealing how neuron-like features affect its output. These interpretable features, which can be understood across languages and modalities like sound or images, are crucial for improving AI safety. Features inside the AI can highlight, in real time, how the model is processing prompts and images. With this information, it is possible to ensure that production-grade models avoid bias and unwanted behaviors that could put safety at risk.
Large language models, such as Claude 3 alongside its predecessor, Claude 2, and rival model GPT-4, are revolutionizing how we interact with technology. As all of these AI models gain intelligence, safety becomes the critical differentiator between them. Taking steps to increase interpretability sets the stage to make AI actions and decisions transparent, de-risking the scaled-up use of AI for the enterprise.
Explainability Lays the Foundation for Safe AI
Anthropic’s paper acts like an FMRI for the “Sonnet” AI model, providing an unprecedented view into the intricate layers of language models. Neural networks are famously complicated. As Emerson once said, “If our brains were so simple that we could understand them, we would not be able to understand them!”
Considerable research has focused on understanding how self-taught learning systems operate, particularly unsupervised or auto-encoder models that learn from unlabelled data without human intervention. Better understanding could lead to more efficient training methods, saving time and energy while enhancing precision, speed, and safety.
Historical studies on visual models, some of the earliest and largest before the advent of language models, visually demonstrated how each subsequent layer in the model adds complexity. Initial layers might identify simple edges, while deeper layers could discern corners and even complete features like eyes.
By extending this understanding to language models, research shows how layers evolve from recognizing basic patterns to integrating complex contexts. This creates AI that responds consistently to a wide variety of related inputs—an attribute known as “invariance.” For example, a chart showing how a business’ sales increase over time might trigger the same behavior as a spreadsheet of numbers or an analysts’ remarks discussing the same information. Thought impossible just two years ago, the impact of this “intelligence on tap” for business cannot be underestimated, so long as it is reliable, truthful, and unbiased…in a word, safe.
Anthropic’s research lays the groundwork for integrating explainability from the outset. This proactive approach will influence future research and development in AI safety.
The Promise of Opus! Demonstrating Scalability
Anthropic’s Opus is poised to scale these principles to a much larger model by proving the success of Sonnet’s interpretability, testing whether these features hold at an even grander scale. Key questions include whether higher levels in Opus are more abstract and comprehensive, and if these features remain understandable to us or surpass our cognitive capabilities.
With evolutions in AI safety and interpretability, competitors will be compelled to follow suit. This could usher in a new wave of research focused on creating transparent and safe AI systems across the industry.
This comes at an important time. As LLMs continue to advance in speed, context windows, and reasoning, their potential applications in data analysis are expanding. The integration of models like Claude 3 and GPT-4 exemplifies the cutting-edge possibilities in modern data analytics by simplifying complex data processing and paving the way for customized, highly effective business intelligence solutions.
Whether you’re a data scientist, part of an insights and analytics team, or a Chief Technology Officer, understanding these language models will be advantageous for unlocking their potential to enhance business operations across various sectors.
Guidance for Explainable Models
A practical approach to achieving explainability is to have language models articulate their decision-making processes. While this can lead to rationalizations, sound logic will ensure these explanations are robust and reliable. One approach is to ask a model to generate step-by-step rules for decision-making. This method, especially for ethical decisions, ensures transparency and accountability, filtering out unethical attributes while preserving standards.
For non-language models, explainability can be achieved by identifying “neighbors.” This involves asking the model to provide examples from its training data that are similar to its current decision, offering insight into the model’s thought process. A similar concept known as “support vectors” asks the model to choose examples that it believes separate the best options for a decision that it has to make.
In the context of unsupervised learning models, understanding these “neighbors” helps clarify the model’s decision-making path, potentially reducing training time and power requirements while enhancing precision and safety.
The Future of AI Safety and Large Language Models
Anthropic’s recent approach to safe AI not only paves the way for more secure AI systems but also sets a new industry standard that prioritizes transparency and accountability from the ground up.
As for the future of enterprise analytics, large language models should begin moving towards specialization of tasks and clusters of cooperating AIs. Imagine deploying an inexpensive and swift model to process raw data, followed by a more sophisticated model that synthesizes these outputs. A larger context model then evaluates the consistency of these results against extensive historical data, ensuring relevance and accuracy. Finally, a specialized model dedicated to truth verification and hallucination detection scrutinizes these outputs before publication. This layered strategy, known as a “graph” approach, would reduce costs while enhancing output quality and reliability, with each model in the cluster optimized for a specific task, thus providing clearer insights into the AI’s decision-making processes.
Incorporating this into a broader framework, language models become an integral component of infrastructure—akin to storage, databases, and compute resources—tailored to serve diverse industry needs. Once safety is a core feature, the focus can be on leveraging the unique capabilities of these models to enhance enterprise applications that will provide end-users with powerful productivity suites.